Search this Site
Culture, Climate Science & Education
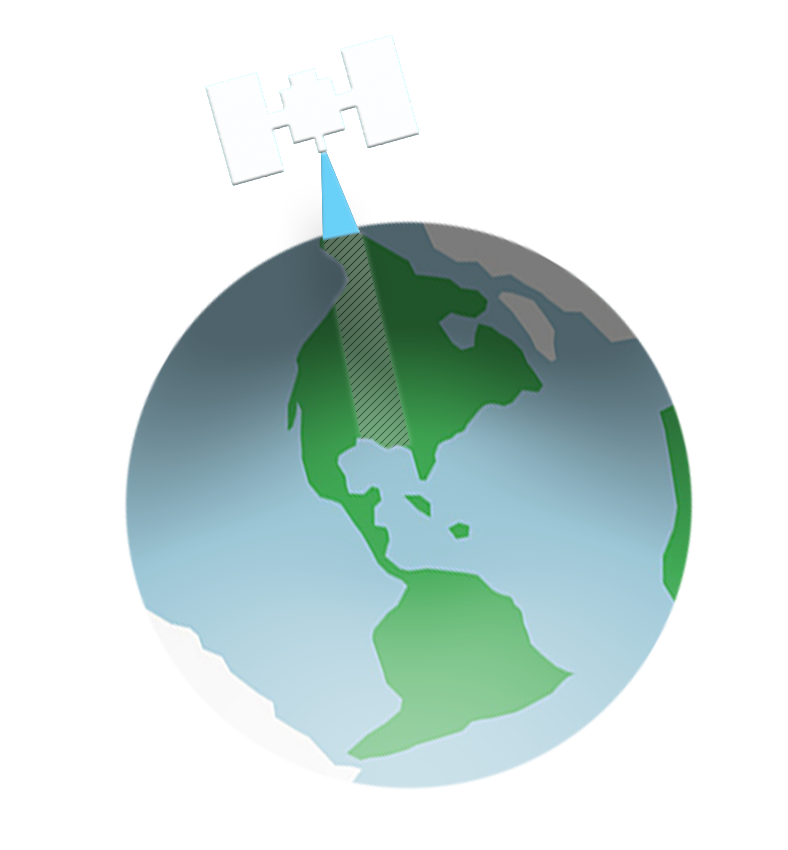
SECTION FOUR
Characteristics of Imagery
Click the Bubbles Above or the Double Arrows () to Explore this Section
A. Introduction
NASA’s fleet of earth observing satellites. Credit: NASA's Goddard Space Flight Center. 2017 April 19.
As you will see though this course, remote sensing allows us to gather an amazing range of information about the natural world. Hopefully we will use knowledge make better decisions regarding how we use, manage, or preserve our limited natural resources.
Remote sensing is part of our toolkit, but what sensor matches what purpose? Just as you would not want to fish for northern pike with a trout fly, you would not get very far monitoring weekly spring green-up with annual aerial photography.
In this section you will learn characteristics of imagery, particularly resolution—spatial, temporal, spectral, radiometric—and positional accuracy. You will then know what aerial or satellite sensor best fits the question or need at hand.
Click the Bubbles Above or the Double Arrows () to Explore this Principle
B. Photographic vs Digital Images
  Click image to expand
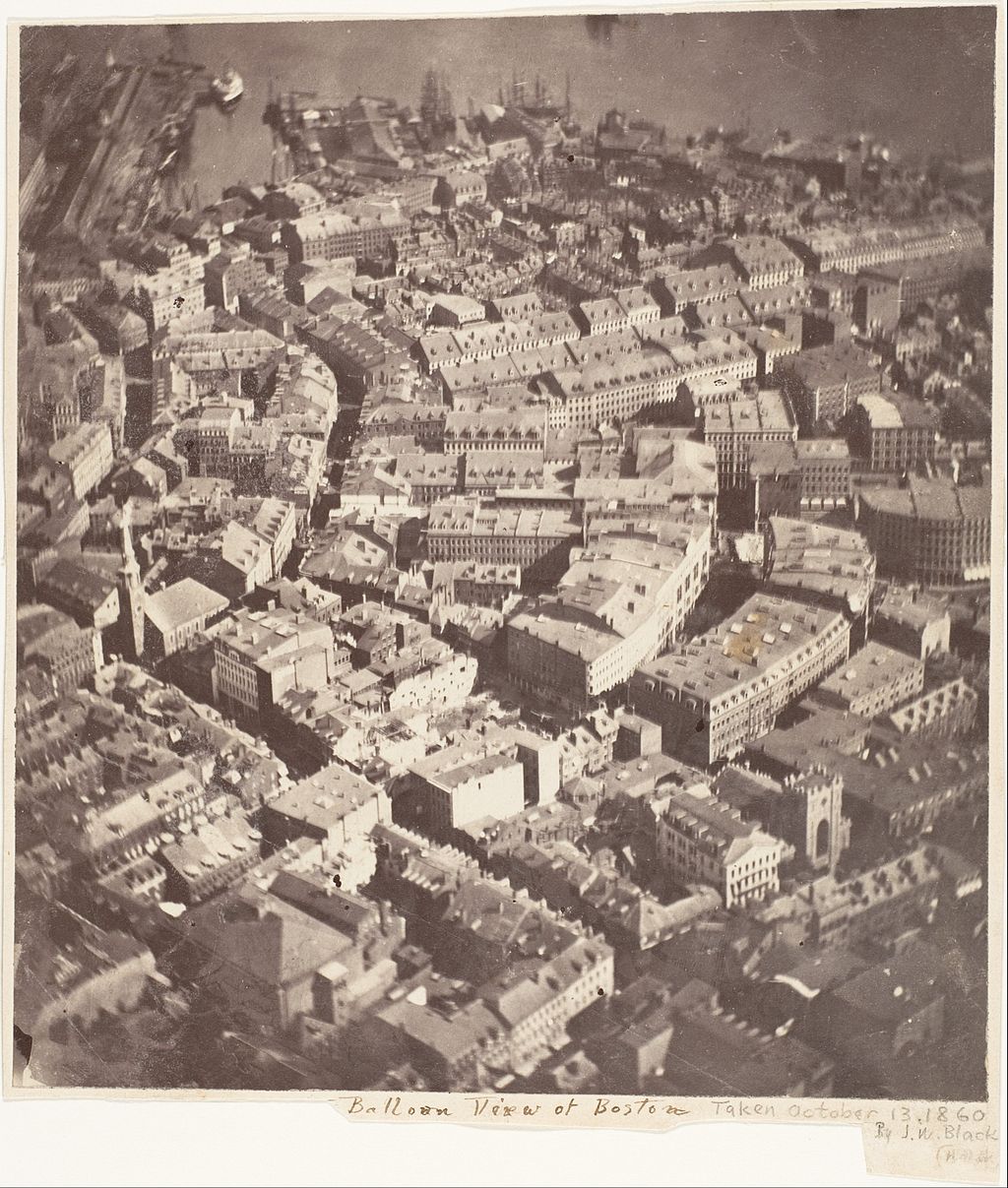
Aerial Photograph over the East Fork Trinity River, TX. USDA AFPO
Images used in remote sensing may be captured on traditional photographic film or, more commonly now, by an electronic sensor. Incremental advances in digital remote-sensing cameras have resulted in the gradual replacement of film with digital imagery.
Photographs are created when light entering a camera activates light-sensitive chemicals embedded in the film’s emulsion layer. The size of these light-sensitive silver halide crystals determine the image’s resolution (graininess). Film’s microscopic photosensitive grains lead to high resolution, an advantage over similarly priced digital cameras (as of 2018).
Large format, specially calibrated “metric” cameras, mounted on aircraft, give us the ability to take measurements from high-resolution photographs.
  Click images to expand

Donald E. Simanek. 2008. Stereoscopy and Illusions. Accessed 2018 Dec 20. http://www.lockhaven.edu/~dsimanek/3d/stereo/3dgallery.htm

Dolores River gorge, Colorado. Stereoscopy significantly enhances our ability to interpret aerial images—and can be used to create elevation maps. Without a stereoscope, the technique for viewing depth is similar to that of Magic Eye®3D posters, where for example, a sailboat seems to emerge from the poster. Source: Bob Stahl. Aerial Photos & Stereo Photography. Accessed 2018 Dec 20. http://www.geocities.ws/potatotrap/tech/aerialx.htm
Analysis with photographic imagery
In regard to the quantitative measurement of spectral signatures (a key feature of modern remote sensing) film-based imagery is more limited than digital imagery. However, much information was (and can still be) collected with photographs through analysis called photogrammetry and photointerpretation.
Photogrammetry refers to measurements such as location, distance, & size of image features. Stereoscopy techniques create 3D visualizations and are used to create terrain & elevation datasets. For fun, click on the button at the bottom of this page to view a gallery of vintage stereo photo pairs. It demonstrates how stereo vision increases detail, depth, and context for interpreting aerial photographs. But before you do, you might click here to see hints on how to view 3D stereo pairs.
Photointerpretation is figuring out “what is going on” in a photograph—identifying features in a photo and determining their significance. This is still very much a part of remote sensing; skilled image interpreters work like detectives, using clues such as object location, size, shape, shadow, tone/color, texture, pattern, height/depth, and site/situation/association.
Antique Stereo View Cards for Parallel Viewing |
Early form of stereoscope. |



























































Click the Bubbles Above or the Double Arrows () to Explore this Principle
C. Digital Images
  Click image to expand

Source: Potash & Phosphate Institute (PPI), ), www.ppi-far.org/ssmg
Digital imagery was developed to overcome limitations of film-photography—such as the ability to precisely calibrate spectral reflectance and to transmit images from space. The first Corona spy satellites (1959-1972) parachuted film canisters back from space; film was developed days or weeks after commencing the launch-recovery process. NASA’s Explorer 6 transmitted the first crude images of earth orbit in 1959, via “slow scan television” technology. The KH-11 U.S. reconnaissance satellites were the first to feature CCD digital sensors in 1976 and revolutionized the ability to deliver real-time imagery from space (Vick 2007).
For decades, aircraft-based imagery used film, due to its higher resolution and lower cost. By 2009—with falling costs of digital sensors and increased demand of “4-band” imagery including visible & near infrared wavelengths—most NAIP aerial image surveys used digital sensors.
Drones and UAVs (unmanned aerial vehicles) are emerging sources of high-resolution digital imagery.
Digital imagery, both aerial- and space-based, will be the focus of this course. Benefits of digital imagery include:
Digital Sensor Design
  Click image to expand

For more information about sensor design, visit the above “Cambridge In Colour” url (brief overview) or the following article link for a detailed overview of current sensors: https://www.intechopen.com/books/multi-purposeful-application-of-geospatial-data/a-review-remote-sensing-sensors
Source: Cambridge In Colour. Copyright © 2005-2018. Digital Camera Sensors. Accessed 2018 Dec 22. https://www.cambridgeincolour.com/tutorials/camera-sensors.htm
Digital sensors capture images with a grid array tiny of light sensitive detectors, or “photosites”. Each photosite corresponds to one image pixel; pixel count is a way to describe camera format size, or image detail. A high-end “large format” sensor array with 17,310 x 11,310 photosite rows/columns contains 195,776,100 pixels—referred to as a 196 megapixel camera. In addition to a “framed” rectangular array of photosites, other design options include “pushbroom” and “whiskbroom” scanners.
To measure reflectance separately for each band, some sensors use color filters over one sensor array, while other designs use a prism to separate wavelengths to separate photosite detector arrays.
Click the Bubbles Above or the Double Arrows () to Explore this Principle
D. Color and False-Color Imagery
Computer displays and television screens create color by blending the primary colors—Red, Green and Blue—this “RGB” system can produce any color. Everyday digital camera (e.g. camera phones) produce one file per picture—the colors have already been mixed.
  Click image to expand

Source: Humboldt State University. http://gsp.humboldt.edu/olm_2015/courses/gsp_216_online/lesson3-1/bands.html
Remote Sensing Multispectral Scanners (MSS) record each band (wavelength range) separately. The result is a separate grayscale image “layer” file for each band. Bands are saved separately so that spectral analysis & image processing are possible. This system also allows us to display “invisible” bands like infrared, because we can display any band as any RGB color.
Normal Color Images
MSS images can be displayed in “normal color” by displaying the red band as red light on a screen, green band as green, and blue band as blue.
Color Mixing
Visit this website and experiment with blending different intensities of red, green, and blue light to create other colors. In this “additive color” system, combining all visible wavelengths creates white light.
Optional Activity: Additive Colors and Color Perception
Go here to explore additive colors and color perception.
View Landsat 8 Band Reflectance in Grayscale & Color
Visit this website to explore Landsat 8 band reflectance by following these steps:
  Click image to expand

In this CIR image, healthy vegetation with access to water (riparian, irrigated) contrasts with dry grasslands. Semi-arid Florence MT, September 28, 2012. Source: USGS NHAP
False Color Images
Displaying colors other than “what they are” creates false color images. To see invisible wavelengths, for example, you could display invisible Near Infrared (NIR) light as Red on your computer screen. That means actual red light reflectance is shuffled and displayed as Green on your screen. Green light reflectance is displayed as Blue. This occupies all the RGB display slots and actual blue light (blue band) is not displayed.
This particular “band combination” is called Color Infrared (CIR). It is commonly used because healthy vegetation stands out from other areas, and water contrasts well with land. Different band combinations highlight different features or phenomenon. Later in this course, you will learn band combinations that highlight snow/ice cover and wildland fire burn intensity.
Band Combinations
Visit this website and follow these steps:
Click the Bubbles Above or the Double Arrows () to Explore this Principle
E. Pixels and Pixel Values
  Click image to expand

Source: Canada Centre for Mapping and Earth Observation, Natural Resources Canada. 2016-08-17. Accessed 2018 Dec 21. https://www.nrcan.gc.ca/earth-sciences/geomatics/satellite-imagery-air-photos/satellite-imagery-products/educational-resources/14641
Remote sensing images are a grid of pixels, just like pictures from a camera phone. Each pixel each contains one value—surface reflectance, averaged over the ground area the pixel covers. Remote sensing cameras are calibrated to a degree that each pixel’s reflectance value is scientific data, not merely a color-value used to create a backdrop image.
A digital image composed of pixels (aka cells) is called a raster. All aerial and satellite images are raster datasets. Some GIS datasets display features with points, lines, or polygons—these are called vector datasets.
Radiometric Calibration
In the last activity, pixel values ranged from 0 – 255. An 8-bit image can distinguish 256 levels of brightness or shades of gray. These non-calibrated pixel values are referred to as raw “Digital Numbers” (DN)—these have not been corrected for the effects of atmospheric absorption, sun angle, sun intensity, etc. Unprocessed DNs can be used for purposes of displaying scenes or some image analysis (“band ratio” techniques cancel out most atmospheric and solar effects).
  Click image to expand

Source: http://gsp.humboldt.edu/olm_2015/courses/gsp_216_online/lesson4-1/radiometric.html. Accessed 2018 Dec 26
Other remote sensing analyses require radiometric calibration—corrections for atmospheric, & solar influences on electromagnetic energy before it reaches the sensor. Raw digital numbers can be corrected to radiance—the radiative energy from each pixel (units of watts per steradian per m2 per μm). Radiance can then be converted to reflectance—the percent of light striking an object that is reflected. Surface reflectance is the “preprocessed” corrected pixel value used to identify features with spectral reflectance analysis.
  Activity Four
(Click to go to Activity Four)
Pixel Reflectance Value
Visit this website and follow these steps:
  Extra Learning
(Click to Learn More)
Click the Bubbles Above or the Double Arrows () to Explore this Principle
F. Spatial Resolution
  Click image to expand

30 vs 2.5 meter Deadhorse Airport North Slope, Alaska.
Source: Alaska Geospatial Council. http://agc.dnr.alaska.gov/imagery.html
The next several pages review aspects of resolution—detail level we can gather from an image. Images differ in resolution spatially, temporally, spectrally, and radiometrically.
The spatial resolution of an image is determined by pixel size—you can’t zoom into a pixel and view additional detail.
Course resolution satellites (e.g. MODIS with 1 km to 500 m pixels) lack spatial detail but can afford a wide image swath every orbit—re-imaging every location on earth every 1-2 days. Moderate resolution satellites (e.g. Landsat 30 m, Sentinel-2) have given us continuous earth coverage since 1972; a strength is landscape-scale change over time. Free, public, high resolution imagery is available from aerial surveys. USDA NAIP, generally 1 meter pixel, 4-band imagery, are re-flown every ~3 years for the continental US.
Commercial satellites offer < 0.5 meter imagery for a price. Drones are increasing used to gather sub-meter custom aerial surveys for purposes such as archaeology, weed management, & natural resource management.
In a 30 meter image, what do you think is the smallest feature you could identify?
The original pixel size at which a digital image is collected is called ground sample distance (GSD). Landsat 8 features 30 x 30 m pixel imagery; the GSD is 30 meters.
Visit this website and compare pixel size for three sensors.
Click all three sensors (display all) and zoom into the smallest tile (IKONOS)
What is the pixel size for this IKONOS image? What is the smallest feature(s) you can reliably identify?
Zoom out so you can see some Landsat imagery around IKONOS. Turn IKONOS on/off. Notice how Landsat generalizes each pixel—each cell may contain both trees, bare ground, and/or road. Zoom out more—what is the smallest feature(s) you can reliably identify with Lansdat? What is the pixel size?
Zoom out again so you can see the entire Landsat image with a little surrounding MODIS. What is the size of a MODIS pixel? Turn Landsat on/off and see how MODIS generalizes reflectance within a pixel.
Zoom out once more, until you can identify features with MODIS—what are the smallest features you can identify?
Click the Bubbles Above or the Double Arrows () to Explore this Principle
G. Temporal Resolution
Temporal resolution is the frequency of image capture. Aerial surveys flown once every 3rd summer could possibly detect change over a decade, however fluctuations within that time would be lost. Earth observing satellites re-image locations daily to bi-weekly—this allows us to see finer detail as to when things happen, such as spring greenup.
Watch the video at right and then answer the following:
Click the Bubbles Above or the Double Arrows () to Explore this Principle
H. Spectral Resolution
  Click image to expand

Spectral bands that selected instruments on Earth-orbiting satellites record. The bandwidths of past, present, and experimental instruments are shown against the spectral-reflectance curves of bare glacier ice, coarse-grained snow, and fine-grained snow. The numbers in the gray boxes are band numbers of the electromaganetic spectrum. The Hyperion sensor records 220 bands between 0.4 μm and 2.5 μm; in the diagram the individual bands are not numbered. Instruments are abbreviated as: ASTER, Advanced Spaceborne Thermal Emission and reflection Radiometer; ALI, Advanced Land Imager; ETM+, Enhanced Thematic Mapper Plus (Landsat 7); MISR, Multiangle Imaging SpectroRadiometer; MODIS, MODerate-resolution Imaging Spectroradiometer (36 bands, of which 19 are relevant to discrimination of snow and ice); MSS, MultiSpectral Scanner (Landsats 1–3); and TM, Thematic Mapper (Landsats 4 and 5)
Sensors with more, narrower bands can see finer details in spectral reflectance curves. Hyperspectral sensors, with hundreds of narrow bands, have high spectral resolution and can detect reflectance changes over small pieces of the electromagnetic spectrum.
Our ability to identify and classify features depends on spectral resolution. Multispectral sensors can separate needleleaf from broadleaf trees—hyperspectral scanners can often identify separate species.
Visit this website and experiment overlaying different sensor bands on different landcovers.
What sensor would reproduce the most accurate spectral reflectance (i.e. have the best spatial resolution)
What sensor has the lowest spatial resolution?
Name two landcover types with very similar reflectance patterns.
Name two types with very different reflectance.
Visit this website and follow instructions to view spectral reflectance of several minerals and landcover types, as viewed from different sensors’ bands.
Click the Bubbles Above or the Double Arrows () to Explore this Principle
I. Radiometric Resolution
  Click image to expand

An 8-bit image, redisplayed at decreasing radiometric resolution (i.e. gray levels). 1-bit imagery only displays two shades, black & white. Original image was 15 meter, panchromatic band from Landsat 7. Credit: Ant Beck, 2012 Dec 7. https://commons.wikimedia.org/wiki/File:Decreasing_
radiometric_resolution_from_L7_15m_panchromatic.svg#file
Visit this website and and read the short article, highlighting sensor improvements from Landsat 1 to Landsat 9
The bottom “Dwell on this” paragraph describes the advantage of higher radiometric resolution—12-bit imagery from Landsat 8 & 9, compared to previous Landsats.
Use the image slider to compare Landsat 8 & 7 imagery.
Write down your observed differences.
Radiometric resolution is the number of brightness levels (i.e. shades of gray) recorded in an image. This is controlled by the number format, i.e. pixel depth, of the image file. Reflectance data in each pixel is a numerical value. Most imagery is delivered in binary “8-bit” number format—numbers are stored as 8-digit strings of ones and zeros. An 8-bit image can display 28 = 256 shades of gray (i.e. brightness levels); zero in binary format is 00000000 and 255 is 11111111. A 12-bit image (e.g. Landsat 8) can differentiate 212 = 4,096 gray levels.
Human eyes can only differentiate between 40 to 50 shades of gray (Aronoff 2005)—why then would earth observing satellites typically use 8-, 10-, or 12-bit encoding and radar systems us 16-bit? Higher bit encoding reduces signal saturation in very bright areas. Terrain may be shaded due to topography or clouds—more graylevels allow us to see detail in dimly reflected areas also.
As with any resolution, higher radiometric resolution demands more file storage space.
Click the Bubbles Above or the Double Arrows () to Explore this Principle
J. Image Geometric Correction
Most imagery you acquire has been preprocessed for optical distortions, so that the location of each pixel is known and the image “sits” on the correct location over a map. At times, such as when using scans of older aerial photographs, you need to perform these geometric corrections yourself.
  Click image to expand

Steps for preprocessing imagery, including georeferencing, radiometric calibration, and topographic correction. Credit: Young et al (2017).
Image Distortion
Remotely sensed images are frequently combined with map data in Geographic Information Systems (GIS). Maps have one constant scale throughout the scene and are drawn from a perfectly vertical “top down” perspective. Maps have been projected—the process of displaying the round earth surface on a flat map.
Image Rectification
A raw satellite or aerial image cannot be simply overlaid on a map—the perspective view of the camera causes displacement and distortion. Locations and objects do “sit” in the correct place and feature not in the image center are viewed partially from the side and lean away. Objects near the edge of an image however are slightly further from the camera than objects at the
“nadir”, directly underneath. This and terrain relief cause distortions in scale on an image.
The rectification process shifts pixel locations to correct image distortion. Rectification may include orthographic correction—using a digital elevation model (DEM) to remove distortion from terrain—the result is called a orthophoto or orthoimagery. Rectification also usually includes georeferencing, which positions the image correctly on the earth’s surface with ground control points.
Click the Bubbles Above or the Double Arrows () to Explore this Principle
K. Resources: Supplemental Materials
Click the Bubbles Above or the Double Arrows () to Explore this Principle
L. References
Aronoff S. 2005. Chapter 4 Characteristics of Remotely Sensed Imagery. In: Aronoff S. Remote Sensing for GIS Managers. Redlands CA: ESRI Press. p. 69-109.
USGS Spectral Library
Kokaly, R.F., Clark, R.N., Swayze, G.A., Livo, K.E., Hoefen, T.M., Pearson, N.C., Wise, R.A., Benzel, W.M., Lowers, H.A., Driscoll, R.L., and Klein, A.J., 2017, USGS Spectral Library Version 7: U.S. Geological Survey Data Series 1035, 61 p., https://doi.org/10.3133/ds1035
Vick CP. Kh-11 Kennan Reconnaissance Imaging Spaceraft. 2007 April 24, Accessed 2018 Dec 21. https://www.globalsecurity.org/space/systems/kh-11.htm.
Young N, Anderson R, Chignell S, Vorster A, Lawrence R, Evangelista P. 2017. A survival guide to Landsat preprocessing. Ecology. 98. 920-932. 10.1002/ecy.1730.